Next Pathway Leverages AI, LLMs To Speed, Simplify Cloud Migration Projects
Next Pathway is bringing AI and LLMs to the complex tasks migrating legacy data warehouses and ETL pipelines to the cloud. IDN talks with CEO Chetan Mathur.
by Vance McCarthy
Tags: AI, cloud, data warehouse, ETL, GenAI, LLM, legacy, Next Pathway, migration, translation,
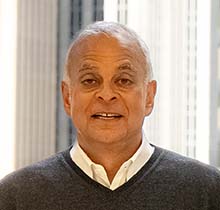
CEO
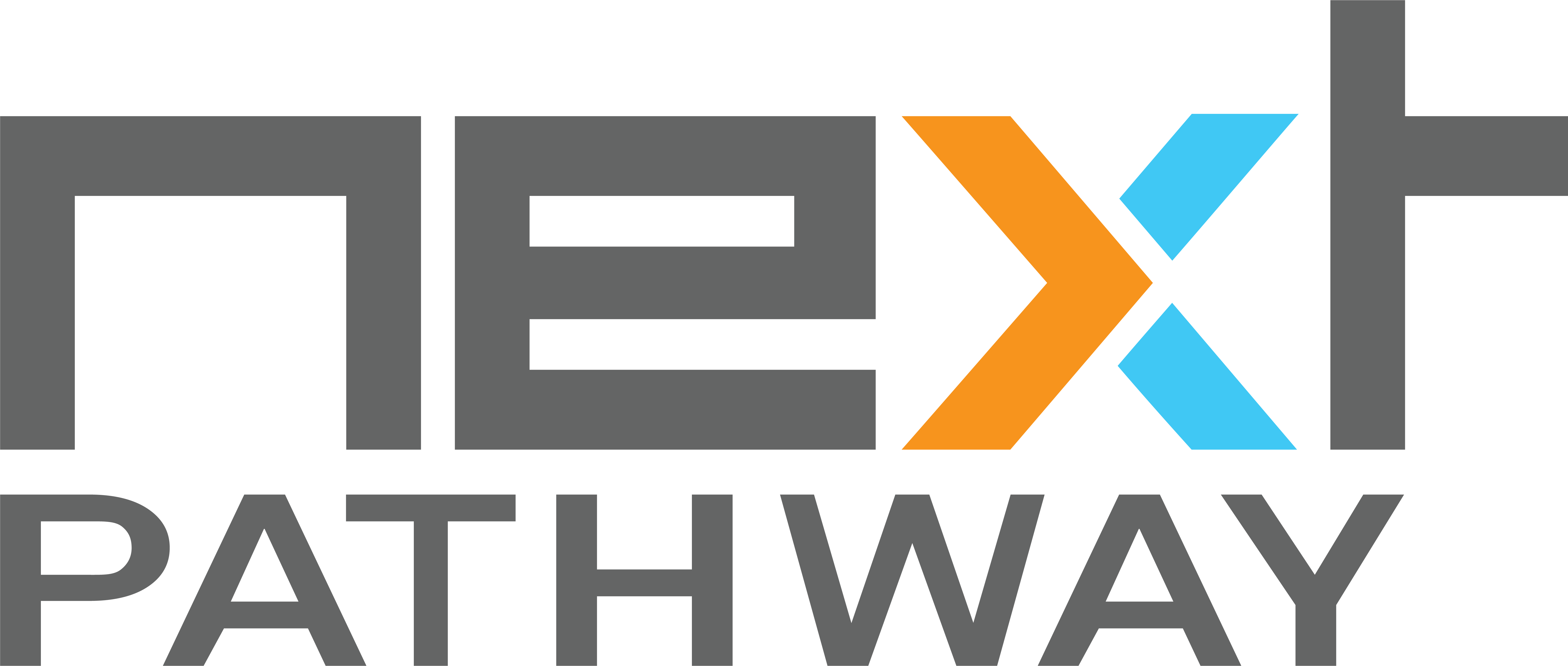
"Our engineering breakthroughs in legacy code migrations make it easier, faster, and cheaper for organizations to AI-ready their data."
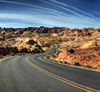
Next Pathway is bringing AI and LLMs to the tasks of modernizing legacy data warehouses and ETL pipelines for cloud and hybrid environment. The result is improved migration planning, translation coverage and testing.
The company is adding GenAI enhancements to its SHIFT Cloud platform, which offers customers automatic code translation for migrating such resources to Microsoft Azure Platform, Microsoft Fabric, the Snowflake Data Cloud and the Google Cloud Platform.
The GenAI-driven features mean more speed, coverage, and accuracy for moving legacy workloads to cloud targets, according to Next Pathway CEO Chetan Mathur. “ "Our innovations are geared to the practical and strategic demands of our customers, we are excited to play a role in helping our clients to enable AI transformation in their businesses,” he said.
IDN spoke with Mathur to explore the architecture and benefits of Next Pathway’s AI enhancements to SHIFT.
Next Pathway’s SHIFT uses AI and LLMs across a couple of key areas, Mathur told IDN.
- Crawling & Translation: Using AI to create “translation prompts” based on prior translated code samples to improve translation performance and coverage.
- Testing: Leveraging AI to generate test data based on the logic within the SQL code and ETL jobs, which creates data, results sets and models that can drive vastly improved results.
"Our engineering breakthroughs are game changers in legacy code migrations. Making it easier, faster, and cheaper for organizations to AI-ready their data," Mathur said.
Next Pathway’s AI/LLMs Improves 3 Critical Areas of Legacy-Cloud Migration
In specific, Next Pathway is using AI to improve a range of complex engineering tasks, especially those critical to 3 fundamental steps in cloud migrations,” according to Mathur.
IDN asked him to share some details about how AI is improving each of these three ‘critical’ areas.
Step 1 - Migration Planning Intelligence.
Next Pathway's has updated its migration planning tool, CRAWLER360, to use AI to predict the optimal migration wave plan based on data dependencies and the criticality and urgency of downstream consuming reports. CRAWLER360 lets users scan and visualize their entire legacy code base to derive the optimal migration wave plan. Further, thanks to AI enhancements, CRAWLER360 also offers improved capabilities to quickly rationalize legacy data warehouses and ETLs to identify which exact workloads can be decommissioned.
Mathur described benefits to customers, and their approach, this way to IDN:
This is a tremendous value-add and a huge improvement to how migrations are executed.
We are leveraging large data sets from prior migrations and using that information we have developed algorithms that can predict the optimal migration path based on the ETL pipelines that are used to ingest data from multiple sources and the pipelines that are feeding end-consuming reports.
For each client migration, we take these models and lay them over the client data to identify patterns. Some clients have preferences and priorities that are predetermined for which applications they want to migrate; however, in most cases clients are unaware of what is actually in their own data warehouse. So, our ‘crawl’ reveals new information about the relationships and dependencies across applications; and informs the most efficient and effective wave plan for the migration.
Step 2 - Code Translation.
As part of the AI-driven update, SHIFT uses AI models to translate transformation logic more quickly within ETL pipelines and SQL code. This means complex ETL platforms (along with SQL Code) can be translated faster than before – and with better accuracy.
The AI-driven increase in speed and accuracy is notable, as Next Pathway’s prior automation was reported in the AWS Marketplace to routinely converts 98%+ of legacy code, with capabilities to quickly get to 100%, while supporting the repoint of ETL and BI tools to the cloud.
We are always looking for faster ways to translate our code. But moreover, in the cases where we identify gaps and need to tackle a problem using manual efforts – our AI models will store all the manual changes we make and recommend an automated solution.
This allows us to take cloud migration teams that are working independently across the globe and ensure that all their manual efforts are reviewed by an AI model.
Step 3 - Testing.
Thanks to AI, SHIFT’s automated code translation also gains better testing. By leveraging the generative capabilities within LLMs Next Pathway has enhanced the accuracy and quality of its code translation.
“Next Pathway's testing capabilities go beyond the generation of vast amounts of simulated test data, to test the logic within the embedded SQL being employed by the ETL jobs,” according to Mathur.
IDN asked whether this focus on AI-driven testing was a request from customers who were looking for smarter or faster automated testing (and pre-testing) to improve the success of their migration projects. Mathur’s reply is revealing and insightful:
Migration projects will eventually become “QA projects” and that is because testing always takes longer than we expect. We are dealing with incredibly complex code and pipelines which can be very time consuming when testing, in particular in the data validation phase. In our efforts to reduce the time burden on our customers and to get them cut over faster, we decided to reimagine how we could use AI to streamline the testing process.
This is the inspiration of how this innovation was developed.
Next Pathway’s Vision for AI’s Future for Legacy Migration
With these AI/LLM elements now incorporated into SHIFT for legacy migration projects, we also wanted to get a glimpse into where Mathur thinks AI will lead Next Pathway in the near future.
Interestingly – but not surprisingly, he told IDN that for Next Pathway, AI will only become a bigger piece of the migration puzzle – not just for implementation and operations, but also early for the scoping and design phases.
The best measure of our success is the time it takes for our clients to cut over to the cloud.
With AI, cut over has been dramatically reduced. We were always efficient in our code translation; but now that we can use AI to create an optimal wave plan and to streamline testing, we can now get clients to the cloud in a matter of a few months. This is a remarkable improvement, because many clients struggled with testing, and it would take them many months after we completed the code translation to get to production.
Companies are investing heavily in preparing their organizations to take advantage of generative AI, which is founded on the principle that everyone can create and consume AI-ready data. However, AI models require vast amounts of data running on a modern data infrastructure. An incredible amount of code and data reside on legacy systems. This institutional knowledge and asset base must be migrated to the cloud in order to take advantage of the data infrastructure and compute power that is only available on modern cloud architectures.
Learn more about Next Pathway’s technologies to automates the end-to-end challenges of migrating legacy apps and workloads to the cloud here.
Related:
- Agentic AI: Unlocking Business Value with the Next Evolution in Enterprise Intelligence
- Zilliz Improves AI-Optimized Vector Databases with More Speed, Scale and Ease of Use
- Informatica, Snowflake Team Up To Speed AI App Delivery; Simplify Data Management and Governance
- Gartner: AI-Augmented Development Hits Radar for 50%-Plus of Enterprises
- OutSystems AI Agent Builder Brings No Code Development to GenAI
All rights reserved © 2024 Enterprise Integration News, Inc.