How Semantic Graph Supports the Quest for True Data Integration and Discovery
With their enterprise data volume and variety growing rapidly, companies want smarter ways to connect and understand all that data – and in context of the business. Stardog CEO Kendall Clark shares why semantic graph data models offer a way forward.
by Kendall Clark, Founder and CEO of Stardog
Tags: API, data discovery, integration, models, non-relational, RDBMS, semantic graph, Stardog,
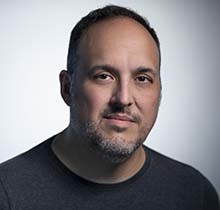
CEO & Founder
"With semantic graph, every kind of enterprise data - including relational and non-relational sources, documents, APIs and legacy systems - can be represented in a standard language."
Any data is potentially of great strategic value, but only if an enterprise can connect and understand that data in the context of what it means to the business itself.
One way growing in popularity is to connect enterprise data using a data model that can represent all aspects - irrespective of location, format, structure, or meaning.
The enterprise needs to connect data in the context of business meaning to really monetize data and transform itself. Data integration systems based on semantic graph data models offer a distinct, new way forward.
Power of Semantic Graph:
How We Got Here & The State of the State
For decades, the only data that really mattered to most enterprises was relational data: rows, columns, tables, few if any nulls, and data sets where the schema didn’t change very often at all. Conventional data integration strategy was developed during this era and it still depends significantly on the relational data model.
As a result, modern non-relational data doesn’t fit well into a relational model.
The rise of NoSQL databases starting about 10 years ago to provide new levels of business value. Storing semi-structured and unstructured data natively unlocked new use cases, and also reduced ETL burdens due to their more flexible schemas. That said, integration capabilities to accompany these new database management systems have been slower to adapt.
As an example, the emerging data fabric space has only just started to emerge to meet the new needs to connect all enterprise data despite the huge growth in data variety.
Enter semantic graph.
With semantic graph, every kind of enterprise data, including relational and non-relational sources, documents, and APIs - even ones in the cloud, as well as different data dialects and structures embedded in legacy systems - can be represented in a standard language. To manage data complexity, the enterprise needs a data model that is powerful enough to represent that complexity.
The representational power of semantic graph lies in its extreme simplicity and consistency: In specific, it represents everything in terms of nodes (i.e., circles) and edges (i.e., lines connecting the circles); then given those nodes and edges names and properties that capture business semantics, using standard data modeling practices.
This power of simplicity allows IT to support queries across relational databases, NoSQL databases, documents, and even geospatial data—seamlessly.
The Name of the Game is Connection
What matters most about enterprise data is “connectedness in context”, that is, understanding how the different parts and pieces of an enterprise’s data landscape relate -- to one another; to business operations; to processes; and even to desired outcomes and objectives.
Relational systems can’t really do that because the relational data model isn’t very good at representing diversity and business meaning. That’s okay, they were created for a different world. Semantic graphs were designed exactly to represent connectedness in context, particularly in complex environments like the Web itself.
So, connectedness as a key, let’s explore how to make it all work – and minimize the implementation burden. Fortunately, there are a growing number of solutions that offer data fabric platforms and incremental implementation patterns and techniques.
Equally helpful, the field is revealing a number of useful best practices that lead to success. Among these are:
- Decide on one or a few specific business questions that need to be answered to create business value.
- Identify key sources of data and metadata, perhaps using existing data catalog(s).
- Build a semantic graph data model MVP that, when queried, answers the questions in step #1.
- Map existing data silos to the data model MVP.
- Build a UX, existing app, or a BI or analytics tool to query the data fabric to get answers to the questions from step #1.
- Increment with new business questions, extensions of the semantic graph data model, and mappings to data silos.
Sematic Graph in a Real World Scenario
To explore some ground-level success, let’s look at financial services.
In this sector, operational risk teams are using semantic graphs to make banks more resilient and adaptable to external and internal risks. One global bank is now using data fabric to analyze 25,000 controls across more than a dozen systems to identify the root cause of risk incidents. This approach allows the bank’s risk teams to see and assess all linked information and dependencies across relevant incident management tools, control registries, and IT asset management systems.
Elsewhere, in the pharma and life sciences sector, teams are using data fabrics to speed up drug discovery R&D. Researchers can link across internal and external data sources—from gene to external world—to see connections in data. With a complete view across topics, projects, and diseases, researchers can more quickly validate drug targets and even reduce the risk of unknown side effects.
We all know that a big data revolution happened in the past 10 years. The key point about that revolution is that it happened first at the data layer itself. Now, that revolution is coming to data integration and data management strategy itself. New innovations like data fabric are built for this brave new data world and use the power of semantic graphs to represent, manage, and ultimately reduce complexity.
Bringing the two together – the data layer and connectedness -- means enterprises can unlock more value from their huge diversity of data silos and data variety. Such opportunities are immense, but are only realizable by considering all of the enterprise’s data in totality.
Kendall Clark is founder and CEO of Stardog, a leading enterprise data company providing an Enterprise Knowledge Graph (EKG) platform powered by smart graph technology.
Related:
- Zilliz Improves AI-Optimized Vector Databases with More Speed, Scale and Ease of Use
- Informatica, Snowflake Team Up To Speed AI App Delivery; Simplify Data Management and Governance
- Gartner: AI-Augmented Development Hits Radar for 50%-Plus of Enterprises
- OutSystems AI Agent Builder Brings No Code Development to GenAI
- Next Pathway Leverages AI, LLMs To Speed, Simplify Cloud Migration Projects
All rights reserved © 2024 Enterprise Integration News, Inc.