Dataiku 7 Aims To Spur AI Adoption with More Collaboration, 'Explainability'
Dataiku is making it easier for data scientists, developers and business users to collaborate on AI and machine learning projects. IDN explores Dataiku 7’s ‘explainable AI’ platform updates with Dataiku’s chief customer officer Kurt Muehmel.
by Vance McCarthy
Tags: AI, cloud, collaboration, Dataiku, elasticity, Git, machine learning,
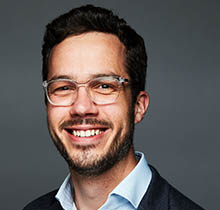
chief customer officer
"The most valuable [AI] applications do not use the most sophisticated models. The challenges are often elsewhere."
Dataiku is shipping an update to its Enterprise AI and machine learning platform to spur more collaboration and make AI/ML projects more accessible.
Dataiku 7 updates the company’s “explainable” AI. The approach comes as a centralized data platform to take teams through crucial steps of what could be called an optimized AI lifecycle -- data prep, model building, AI deployment, management, analytics and full-blown enterprise AI.
Dataiku CEO, Florian Douetteau shared how important coloration has been to the company’s platform from the beginning.
“Collaboration has been at the core of Dataiku since our founding in 2013, and with Dataiku 7, we’re continuing to add features that deepen our philosophy to effectively democratize AI in the enterprise,” he said in a statement. “Dataiku 7 is our second consecutive product release that expands features for explainable AI, a critical component for organizations across industries to succeed and understand the impact of their AI model outcomes.”
The most recent release, Dataiku 7 sports several features to promote more collaboration among stakeholders across multiple disciplines. They include:
Git for Better Coder Collaboration: With the enhanced Git integration in Dataiku 7, data scientists (or other code-first users) can now create, delete, push, and pull Git branches directly from Dataiku. This brings significant efficiency gains, as coders can easily duplicate projects to sandbox changes, leaving the original project unaffected. Once the iteration on the duplicate project is complete, changes can be seamlessly merged back to the original project (with all changes tracked in Git).
Support for Advanced Statistical Analysis: Statisticians can now use Dataiku to perform advanced statistical analysis in the familiar worksheet-and-cards format. This will make it easier for statisticians, data scientists, and analytics teams to collaborate. In the past, advanced statisticians were relegated to siloed tools with no visibility for non-statisticians. This ‘blind’ approach caused bottlenecks and undue delays in governance and AI project deployment.
Upgraded Integration with Microsoft 365 Services, including Microsoft Teams, SharePoint, and OneDrive. This update enables customers using Teams to directly track and share changes made to their AI/ML projects. “Dataiku understands that organizations need to leverage information from multiple sources and create actionable insights to democratize AI projects across their business. Our latest integrations with Microsoft signify a major step toward expanding our platform.” Douetteau said.
Labeling Plug-in for Active Learning: Properly labeled data is a prerequisite for deriving quality insights from machine learning models. It is also a prerequisite to visibility, which, in turn, drives deeper collaboration. Dataiku 7's new plug-in lets users mark data quickly and make data collection less tedious and time-consuming. It brings active learning to labeling while keeping a human-in-the-loop approach. It uses a suite of Dataiku web apps to ease the labeling process, whether data is tabular, images, or even sound.
Why Dataiku Says Collaboration is Key to AI Success
Dataiku execs say that fragmentation across the data science ecosystem is one of the leading causes of why AI/ML projects fail. Dataiku’s chief customer officer Kurt Muehmel put it this way.
“There is no one, single magic bullet. Functionality like low-code, automation, and model repositories are all part of the equation, but there’s more to it,” Muehmel told IDN. “Dataiku provides a single platform that combines necessary functionality, bridges multiple data environments, and enables broad collaboration across different skill sets.”
This misperception of the understanding of how teamwork can be to an AI project can lead to the failure of some AI projects, he added. “There is a real gap between the value that some companies are getting out of their AI/ML efforts, what others say they are getting out of them, and what still others wish they were getting out of them.
“What’s needed is a common, coherent platform that brings together all of the different functionality, sources of data, and--most importantly-- the people required to build and deploy what a given company needs.
Muehmel shared a customer example of Dataiku's multi-discipline, collaborative approach:
One of Dataiku’s largest customers is a major aerospace company. The diversity of skills in their teams is immense: from PhD-level data scientists straight out of academia, to business analysts who have been with the company for 25+ years, to shop floor supervisors with machine oil on their hands. All have a valuable contribution to make to the AI/ML process.
Dataiku enables that collaboration.
The relatively few data scientists use Dataiku to source and prepare data and test multiple ML approaches. Some may use Dataiku’s AutoML capabilities like feature generation and grid search to accelerate their work and frequently will work with their preferred programming language, whether Python, R or Scala, directly within Dataiku.
Once they’ve built out a model, the data scientists can then package it and deploy it to the broader population of analysts or technicians, either as the backend to another system via an API, or by wrapping it up as a no-code plugin that an analyst can then reuse, applying it to a new source of data or a new business question.
All of this requires strong collaboration and knowledge of the management framework, which Dataiku also provides. This enables a user in Hyderbad to discover and reuse work from a colleague in Hartford. All of the individual functionality is useful, necessary even. But taken together, the whole is really greater than the sum of its parts.
In a recent Q&A from the Dataiku blog, Douetteau further explained why such cross-discipline collaboration on AI is showing to be especially valuable in 2020.
More than ever, organizations need collaboration between IT and business profiles as well as actionable ways to bridge the gap and scale their AI projects without running repetitive processes, having clearly documented standards for AI within their organization, or easily being able to access a workflow they’re involved in.
Traits like cross-team communication, workflow reusability, and a collaborative, end-to-end platform that is accessible to all team members are essential to generating true business impact with data science and machine learning.
Other notable features in Dataiku 7 include:
Advanced Prediction Explanations: Traditionally, machine learning models do not include insights into why or how they arrived at an outcome, making it difficult to objectively explain the decisions made and actions taken based on these models.
Prediction explanations in Dataiku open the black box by describing which characteristics, or features, have the most significant impact on a model’s outcomes. Dataiku 7 includes both row-level prediction explanations in output datasets as well as interactive visualizations of individual prediction explanations.
More Elasticity with Kubernetes: Dataiku 7 expands on the managed Kubernetes cluster capability from Dataiku 6 by allowing users to now run web apps on Kubernetes clusters. This enables more concurrent users and a fast, flexible execution backend for resource-heavy AI deployments.
Dataiku’s latest release also sports a dedicated EDA interface for statistical analysis, and row-level explainability to promote white-box AI.
In AI/ML: Don’t Sweat (Just) the Model – Get Real Production Data
Muehmel also noted the key to AI success is to focus on the proper things.
Unfortunately, with so many shiny objects floating around in AI projects, that can be difficult for many companies. He described it this way to IDN:
“If we’re looking for broad patterns, I would say that often, the most valuable applications do not use the most sophisticated models. The challenges are often elsewhere - in data access and preparation, model deployment, and overall ML governance,” Muehmel said.
“This is not to minimize the importance of having a high-performing model, but to emphasize the importance of everything else that’s required for it to actually deliver value to the company. While a perfectly tuned model trained on sample data sure looks nice in a notebook on a laptop, it can’t shake a stick at a basic model trained on production data, serving real-world needs day in and day out,” he added.
Readers can get started with a free edition of Dataiku here.
Related:
- Informatica, Snowflake Team Up To Speed AI App Delivery; Simplify Data Management and Governance
- Gartner: AI-Augmented Development Hits Radar for 50%-Plus of Enterprises
- OutSystems AI Agent Builder Brings No Code Development to GenAI
- Next Pathway Leverages AI, LLMs To Speed, Simplify Cloud Migration Projects
- Gartner Says Only 12% of Infrastructure & Operations Leaders Exceed CIO Expectations
All rights reserved © 2024 Enterprise Integration News, Inc.