The Data Value Chain: Steps for Monetizing Your Data
In today’s data-driven enterprise, the mad dash to capture and analyze mountains of new data can overwhelm both IT and the business. Information Builders’ Rado Kotorov shares the secrets of a Data Value Chain, a methodology to turn any feelings of data chaos into an easy-to-follow roadmap to profits and ROI.
by Rado Kotorov, VP of Marketing Strategy and Chief Innovation Officer, Information Builders
Tags: analytics, data, data quality, decision management, Information Builders, integration,
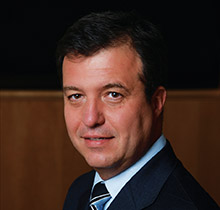
VP of Marketing Strategy
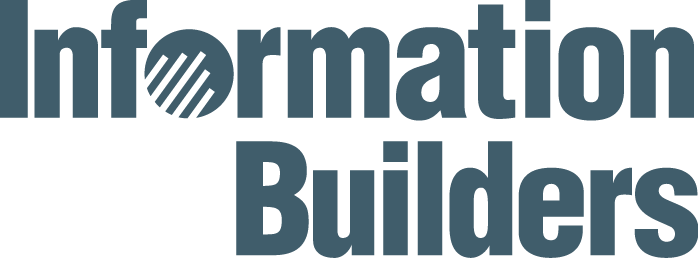
"The ‘Data Value Chain’ guides companies through transforming raw data into a refined product."
Integration & Web APIs
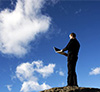
We’ve all heard the analogy: data is the new crude oil. But we rarely focus on the “crude” aspect, which refers to a resource that, without refining, is useless.
Taking the leap from data collection and analysis to using data to make a real difference to the business’s bottom line is a challenge for many organizations. The key is having a ‘Data Value Chain,’ which can let companies tap into data as a new revenue stream or to reduce costs.
The most important step in effecting significant, organization-level change is that you must have a methodology in place that offers checks and balances to make sure that progress is not only continuing, but is doing so in the correct direction. To be effective, this methodology must also be easily understood and embraced among all stakeholders across the enterprise.
The ‘Data Value Chain’ borrows key elements from manufacturing and other industries that convert raw materials into finished goods. This step-by-step approach guides companies through transforming raw data into a “refined product.”
The 5 Key Links in the Data Value Chain
Here are the five fundamentals to the ‘Data Value Chain’ approach. Follow them, and we think you’ll find you have an easy-to-adopt methodology:
1) Data capture
There are a multitude of advanced quality methods to collect and store data from various relational and non-relational sources, as well as data streams. These may be more sophisticated than drills used in oil production, but the idea is the same. The key challenge of data capture is the variety and volume of data that can be captured. This poses a question about how to store this data, but more importantly how to process it for storage in such a way so that it is ready for analysis. Most companies throw the data into data lakes, but then it takes months for the users to get such data ready for analysis. The value of data is perishable. If you do not make it ready for analysis as soon as you capture it, the opportunities may disappear by the time it’s analyzed. Big data presents actionable opportunities now!
2) Data quality and integration
Once collected, data must be organized so that all the resources and components can be assembled into meaningful data units. Consider the various channels through which retail brands interact with customers: in-person, online, via social media, and so on. Given the various points of interaction with a single customer, there is a significant need for all the data to be integrated into a single view or “golden record” of that individual. Only then can accurate segmentation, cross-selling, and up-selling occur. Key in enabling this are proper data quality, governance and MDM (master data management), driven by the need for faster and more accurate decision making. Put plainly, incorrect data leads to wrong decisions. Ungoverned data is frequently unutilized or incorrectly utilized data. Not surprisingly, not much value can be derived from the data assets without governance. We’ve been talking about “360-degree views” of the customer for years, but how can you get such a view without MDM—in other words, without having consistent records of your customers across all channels?
3) Data enrichment
Frequently, organizations acquire external data and append it to their own records. Using the retail example, adding psychographic data—data about preferences and traits—is often useful for better understanding the customer. Combined with customer data, it provides marketers with a better picture of customers’ motivations, allowing them to develop more effective programs and offers.
These first three steps create a unit of data that is ready for analysis. The more complete the unit of data, the more insights can be derived from it. Data that is not used for analysis is waste, like a byproduct of refined oil.
The Data Value Chain. Be sure to follow the entire path!
4) Analytics
Once data units are established, organizations can begin to extract insights on what has happened in the past and what is possible moving forward. Analysis may reveal trends that can be capitalized on, bring to light hidden costs, or reveal new sales and revenue opportunities. Increasingly, organizations use data enrichment to understand why things happen or why consumers behave in certain ways, and with these insights, they create new revenue streams.
There are a number of considerations that must be made along the way. A company has to decide on the type of analytics they want to go after: descriptive, diagnostic, predictive and prescriptive. It also must decide on the presentation layer of analytics, how it will be communicated to end users: via a written report, interactive dashboard, analytical visualization, info application, etc.—or some combination of these. One type of presentation does not fit all use cases. Finally, the delivery vehicle for that information is important—will it find its way to the user through an online portal, a third-party application, embedded in a third-party application or some other way? Each of these three dimensions of analytics are critical parts of the planning process, as they affect how efficiently analytics can be used in decision-making.
5) Monetization
With analysis complete, you now have a data asset, which has the potential to be monetized. The insights—or “aha!” moments—derived from it point toward how those assets can be properly utilized and allow organizations to discover new opportunities, but by itself, insight is not sufficient for monetization. The opportunity that is illuminated needs to make its way into the market somehow, either by operationalizing it or making it available to external parties.
Real-World Benefits of the Data Value Chain Approach
The most notable beneficial outcome from the Data Value Chain is that it supports operational decision making.
The first way to monetize data and analytics is via internal, easy-to-use apps that support decision making within the organization. Those apps are intended to drive fact-based decision making, in turn, changing employee behavior and driving higher levels of performance. These self-service apps are drastically different from traditional business intelligence software. The goal is not to enable employees to perform analysis themselves, but rather to provide factual answers to business questions quickly, based on the user’s role. Therefore all the analysis has to be built into the app.
Consider Expedia.com; the site democratized travel booking by allowing its customers to acquire information about trips and prices quickly and without any need for training. This is a self-service model that others would be wise to imitate. Most organizations do not know how to leverage the insights they’re procuring to build an operational application, and thus are missing out on a significant opportunity.
Beyond this, the Data Value Chain makes data more consumable – and more readily puts data in customers’ hands. Packaging information as a consumer product is the second way to monetize data. We call this the consumerization of analytics. To do this though, organizations must make exceptional ease of use a priority.
Some organizations distribute great volumes of data to consumers in static PDF documents (like a financial statement), but in that format the consumer is powerless to do pretty much anything with the data. This is another missed opportunity. Providing in-document analytics that allow customers to filter information or easily manipulate the data empowers the consumer to make better decisions about their personal business, which in turn creates more revenue opportunities for the organization. For example, when utility companies distribute interactive statements, their customers can make “what if” analyses and decide whether to buy smart meters.
Monetizing Data Also Requires a Culture Change
The transition from data collection to monetization also requires a cultural change. This may be difficult, but it’s crucial to the success of an organization-wide initiative. Think, for example, of the omnipresence of taxi companies up until a few years ago. Major taxi companies have dispatch software in place to map the location of their cars, but they didn’t think to use this data in innovative ways—yet Uber did. It was an industry outsider—a startup with zero cars, but with a culture that promoted the value of data.
Thus, the first step in this is to instill in stakeholders that the Data Value Chain is not only about insights. You must take the next step and follow-through. If you don’t act on insights you glean from analysis, and monetize data, other companies will. It takes a methodology like this—and an organization-wide commitment to it—to remain ahead of the competition.
As vice president of marketing strategy and chief innovation officer for Information Builders, Dr. Rado Kotorov works every day to help make BI and business analytics more accessible, intuitive, and collaborative. He is also a published author on emerging technologies and knowledge management (KM) issues.
Related:
- Agentic AI: Unlocking Business Value with the Next Evolution in Enterprise Intelligence
- Zilliz Improves AI-Optimized Vector Databases with More Speed, Scale and Ease of Use
- Informatica, Snowflake Team Up To Speed AI App Delivery; Simplify Data Management and Governance
- Gartner: AI-Augmented Development Hits Radar for 50%-Plus of Enterprises
- OutSystems AI Agent Builder Brings No Code Development to GenAI
All rights reserved © 2024 Enterprise Integration News, Inc.