Integrating Gartner’s “Top 10 Analytics Trends for 2020” Into Your Data Analytics Lifecycle
In 2020, data analytics will explode with utility and adoption, according to Kaycee Lai, CEO of Promethium. His IDN post focuses on the synergy among three of Gartner's top analytics trends – ADM, NLP and augmented analytics. His perspective come as the founder of a context automation start-up.
by By Kaycee Lai, CEO & founder, Promethium
Tags: analytics, ADM, data management, deep learning, Gartner, NLP, Promethium,
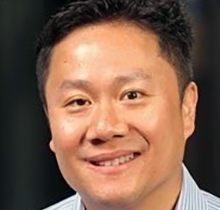
CEO & founder

"Augmented Analytics is the perfect discipline to join with the Gartner’s other two trends - Augmented Data Management and Natural Language Processing."
To help enterprises navigate the word of analytics in 2020, Gartner recently issued its Top 10 Analytics Trends for 2020. To me, three of them represent a synergy with potential to move the industry forward in adoption and utility of analytics -- by leaps and bounds.
In this post, I’d like to examine the impact of three of Gartner’s trends:
- Augmented Analytics,
- Augmented Data Management (ADM), and
- Natural Language Processing (NLP)
As I hope to illustrate, taken together they represent a movement toward what I like to call end-to-end “democratized” analytics, which will make it feasible for all organizations to become truly data-driven – even those without deep pockets or skilled PhDs on staff. The key will be for enterprises to integrate them together so they will support an overarching systemized approach to analytics.
Let’s have a closer look at how these technologies complement each other.
Augmented Analytics: Speeding Time to Insight
Augmented Analytics enhances and accelerates data storytelling and critical thinking, using machine learning (ML) to improve the ease, scale and versatility of analytics projects. It does so by automating data preparation, sharing and model development in ways that allow organizations to handle larger data sets, work more quickly from raw data, present clearer results, and find hidden patterns based on more statically significant findings.
Augmented Analytics is about improving the user experience to the point where it’s possible to analyze data with minimal time and technical chops. Where data scientists now spend weeks cleaning and prepping datasets for analysis, Augmented Analytics allows organizations to enter raw data into platforms which in turn leverage ML to ready it for analysis.
Augmented Analytics boils down to speed. Properly implemented, this trend is key to solving a major issue--the delay of data prep. Lengthy data prep, in turn, also lengthens the process of data mining, often by orders of magnitude. However, augmented analytics opens the possibility of skipping several very time-consuming manual steps.
Moreover, it affords agility. Where data scientists currently rely heavily on intuition and experimentation to identify trends, ML can accelerate this by automatically identifying statistically significant items, which may warrant further analysis.
As I’ll explain below, Augmented Analytics is also the perfect discipline to join with the Gartner’s other two trends, which we’ll now discuss. Since you can’t analyze data that you can’t find, this won’t be possible without Augmented Data Management (ADM), which simplifies locating data across complex environments. Furthermore, NLP opens the door to interacting with data in more intuitive ways, without complex coding.
ADM: Augmented Data Management Gets Straight to Business
ADM overlaps with Augmented Analytics in many respects. But generally, it takes aspects of data management--from database admin to disaster recovery--and augments them with machine learning, automation and AI.
Presently, this labor-intensive work means that only 20 percent of data scientist and engineer time is spent analyzing data--the other 80 percent is spent wrangling and babysitting it. ADM lets them get straight to the analytics.
Why haven’t these tasks already been automated? Because, as Forrester’s Michelle Goetz has pointed out, they require technical and subject matter expertise, and include such processes as:
- Labeling, classification, and tagging
- Gauging the value, completeness and trustworthiness of data
- Determining whether data complies with industry regulation and company policies
- Matching and merging records across silos, platforms and tools for a single version of the truth
- Managing and enforcing metadata, or the ‘data that describes the data’, for classification, history and other purposes
Machine learning allows such tasks to be performed at scale and can even go a step further to infer metadata based on user behavior in past interactions with the data. The next slew of advances in this area in no small part involve NLP, which promises to add a layer of context and meaning to data that makes it actionable for the non-technical.
NLP: Democratizing Data Insight
We communicate with computers on their terms--in code. NLP reverses this, allowing humans to speak to computers in human language. Machine learning models, in contrast to traditional hard-coded programs, deal with probabilities vs. strict rules, allowing them to deal with unfamiliar input and infer meaning based on previous data. Now deep learning, which mimics the human brain, is taking things further with programs that can tune to the nuances of human speech.
With standard technology in data management/analytics, if you want to retrieve data for a specific problem, at best you might rely on keyword search, which of course assumes the right keywords are attached to the dataset. At worst you’ll need to know the exact name of the tables you’re looking for. Either way, it’s a productivity-killer. With NLP, however, you can locate data by posing the business question directly to the computer, which then locates the right tables.
Integrating NLP, Augmented Data Management and Augmented Analytics
While it may be true that organizations will have fancier tools that in theory make the process of data mining much easier, the fact is that they won’t deliver the intended value unless your enterprise-wide analytics conforms to a structured process.
To avoid the “collection of tools with no direction” scenario, don’t think about Augmented Analytics, ADM and NLP as distinct components. Rather, consider how they support an overarching analytics system.
One example we like is CRISP-DM, which offers a six-phase blueprint for a data mining project:
Phase One: Business Understanding. Determine the business objectives, assess the situation, take stock of available data, set your project goals, and make a game plan. As the advice of business domain experts is critical here, at this phase you view ADM and NLP, as tools that allow their hands-on involvement at the start, breaking down some of the walls that have separated them from data analysts.
Phase Two: Data Understanding. Here the analyst takes a high-level look at the data for initial insights. The aforementioned technological innovations are particularly important here, as they will enable analysts to work more closely with business experts, who can advise on the meaning behind the patterns, as well as what is relevant, and what might be anomalous or noise. All of this will be crucial in forming a hypothesis for the analytics project.
Phase Three: Data Preparation. Datasets come with numerous problems--from formatting to missing values--that make them impossible to run through computer models. Here Augmented Analytics can play a critical role by automating outlier detection and imputation of missing values, and other cleaning functions.
Phase Four: Modeling. This is where you decide what kind of algorithms or models will yield the answers you need. It’s also where analytics turns into Greek for most business experts. While some of this can’t be avoided, Augmented Analytics can help in more quickly identifying the structure of the data and more easily implementing the correct model.
Phase Five: Evaluation. At this point the team will evaluate the model and pose a critical question: can it be repeated and integrated into business processes? For effective implementation, new data--not just data used in the pilot study--has to be readily available on an ongoing basis. This takes us back to several key aspects of ADM, such as the matching and merging records across data silos, and managing/enforcing metadata, which are key to making this happen.
Phase Six: Synergy. In this final phase, your analytics team works with the software development team and business domain experts to bake the new capabilities into your architecture and processes. With Augmented Analytics, ADM and NLP, this synergy between three areas of business that often don’t understand each other becomes much more achievable.
Kaycee Lai is CEO and founder of Promethium. He has led global operations and product management for a number of Fortune 500 and up-and-coming companies for 20 years, including Waterline Data, Virsto Software and EMC. He also has worked at Microsoft and the U.S. Federal Reserve.
Related:
- Zilliz Improves AI-Optimized Vector Databases with More Speed, Scale and Ease of Use
- Informatica, Snowflake Team Up To Speed AI App Delivery; Simplify Data Management and Governance
- Gartner: AI-Augmented Development Hits Radar for 50%-Plus of Enterprises
- OutSystems AI Agent Builder Brings No Code Development to GenAI
- Next Pathway Leverages AI, LLMs To Speed, Simplify Cloud Migration Projects
All rights reserved © 2024 Enterprise Integration News, Inc.